Machine Learning Demo
Example using different classifiers.
K-class classification data of 2D points (training data)
K = 3; % number of classes (pick a number between 2 and 6)
[X,Y] = cv.createConcentricSpheresTestSet(200*K, 2, K);
Y = Y(:);
create grid of points to cover domain of data (testing data)
mn = min(X); mx = max(X); N = 100; [X1,X2] = meshgrid(linspace(mn(1),mx(1),N), linspace(mn(2),mx(2),N)); X1X2 = [X1(:) X2(:)];
some colors for plots
if true clrBG = [1.0 0.7 0.7; 0.7 1.0 0.7; 0.7 0.7 1.0; ... 0.7 1.0 1.0; 1.0 0.7 1.0; 1.0 1.0 0.7]; clrFG = [1 0 0; 0 1 0; 0 0 1; 0 1 1; 1 0 1; 1 1 0]; % 'rgbcmy' else clrBG = brighten(lines(6), 0.7); clrFG = lines(6); end
different machine learning models
models = {'ANN_MLP', 'DTrees', 'KNearest', ... 'LogisticRegression', 'NormalBayesClassifier', 'SVM'}; figure('Position',get(0, 'DefaultFigurePosition').*[0.5 0.5 1.8 1.8]) for i=1:numel(models) % create ML model switch models{i} case 'ANN_MLP' obj = cv.ANN_MLP(); obj.LayerSizes = [2 4 4 K]; obj.setActivationFunction('Sigmoid', 'Param1',1.0, 'Param2',1.0); obj.TermCriteria.maxCount = 2000; obj.TermCriteria.epsilon = 1e-4; case 'DTrees' obj = cv.DTrees(); case 'KNearest' obj = cv.KNearest(); case 'LogisticRegression' obj = cv.LogisticRegression(); case 'NormalBayesClassifier' obj = cv.NormalBayesClassifier(); case 'SVM' obj = cv.SVM(); end % train if strcmp(models{i}, 'ANN_MLP') % ANN_MLP doesn't handle categorical data, we use 1-of-N encoding YY = bsxfun(@eq, Y, 0:(K-1)); % [Y==0 Y==1 .. Y==(K-1)] else YY = int32(Y); end tic obj.train(X, YY); toc % predict YYhat = obj.predict(X1X2); if strcmp(models{i}, 'ANN_MLP') [~,Yhat] = max(YYhat, [], 2); else Yhat = int32(YYhat); end % plot predictions and actual classes subplot(2,3,i) imagesc(X1(1,:), X2(:,1), reshape(double(Yhat)+1, size(X1))) hold on if mexopencv.isOctave() %HACK: GSCATTER not implemented in Octave scatter(X(:,1), X(:,2), 6, clrFG(double(Y)+1,:), 'filled') else gscatter(X(:,1), X(:,2), Y, clrFG, '.', 6, 'off') end hold off colormap(clrBG(1:K,:)) axis equal, axis([mn(1) mx(1) mn(2) mx(2)]) title(models{i}, 'Interpreter','none') end
Elapsed time is 0.053490 seconds. Elapsed time is 0.002736 seconds. Elapsed time is 0.001419 seconds. Elapsed time is 1.274223 seconds. Elapsed time is 0.003751 seconds. Elapsed time is 0.007012 seconds.
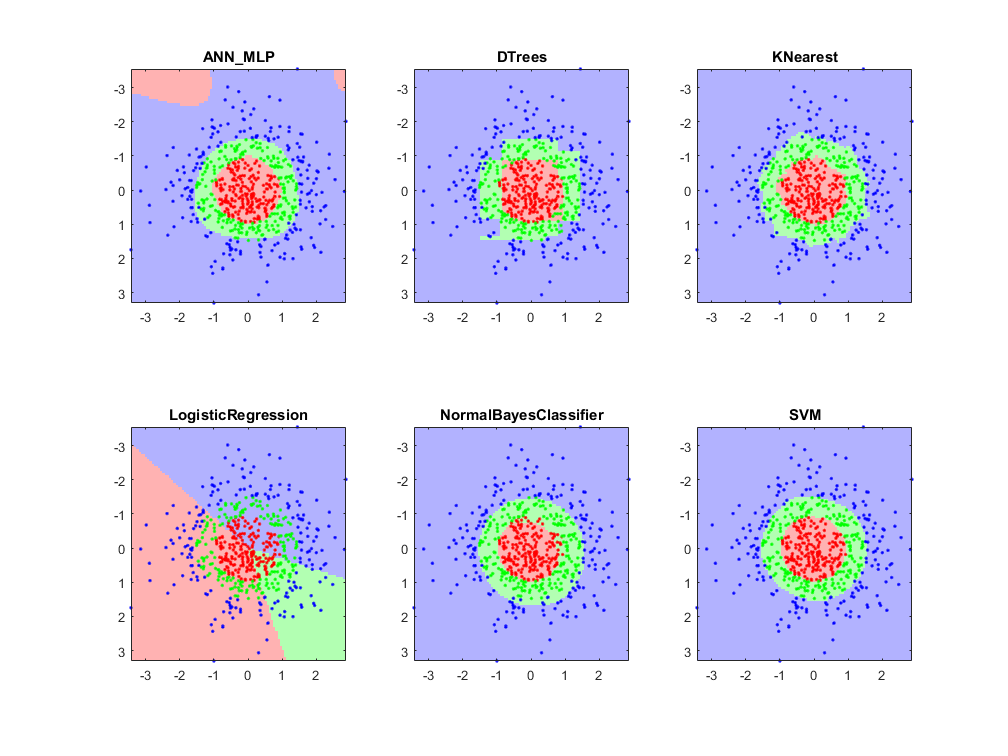